Multi-fidelity surrogate models
Principal investigator: Imad Abdallah
Description
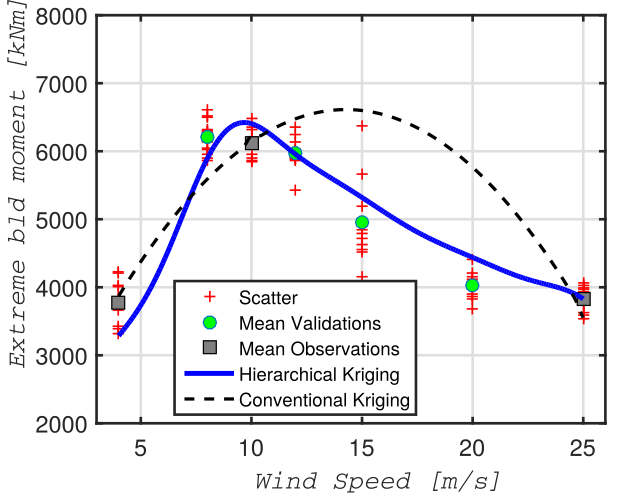
Engineers use multiple computer simulators to predict and compare a certain quantity of interest. The project examines how to fuse predictions from multiple simulators using the Kriging surrogate modelling technique (Abdallah et al., 2015). In particular we investigate a hierarchical Kriging approach (Han and Görtz, 2012). The idea is that fusing predictions from multiple simulators in the early stages of the conceptual design of a structure or a mechanical system results in reduction in model uncertainty. As an application we fuse the output from two aeroelastic simulators of a wind turbine.
The elements of a wind turbine aeroelastic simulator include models to simulate the turbulence wind field, offshore waves, currents and ice drifts, terrain roughness and soil. These external conditions are translated into loads using aerodynamic, hydrodynamic, soil dynamic and earthquake models. Finally the external loads are applied on the wind turbine structure through the actions of a control system and translated into structural reactions using finite element or multibody dynamics models. We implement a hierarchical Kriging technique to predict the extreme response in the presence of non-stationary noise in the output (i.e. the magnitude of noise varies as a function of the input variables) in the case when the low and high-fidelity aeroelastic simulators of the same wind turbine are implemented. The figure shows a comparison of the hierarchical Kriging and conventional Kriging surrogate models of the high-fidelity extreme blade root flapwise bending moment.
References
Han, Z.-H. and S. Görtz (2012). Hierarchical Kriging model for variable-fidelity surrogate modeling. AIAA Journal 50, 1885–1896.
Berchier, M., Multi-fidelity Surrogate Modelling with Polynomial Chaos Expansions, MSc Thesis, ETH Zürich (2016).