Dimensionality reduction for surrogate models in ultrahigh dimensions
Principal investigator: C. Lataniotis
Description
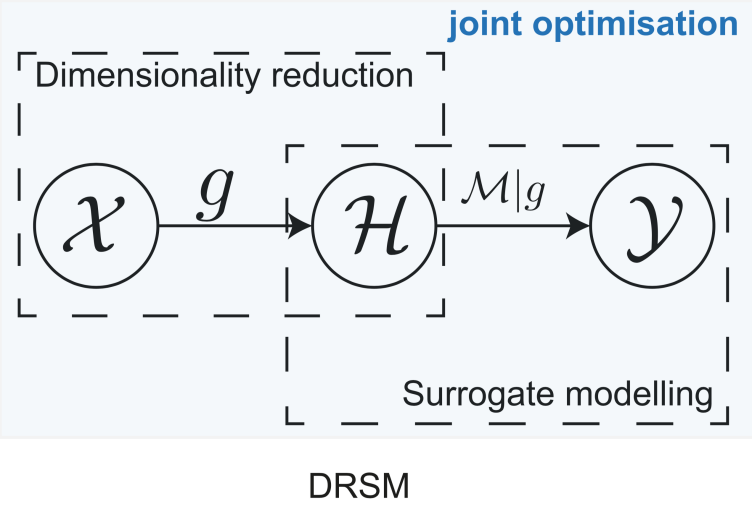
Surrogate models are often used in the context of uncertainty quantification for substituting computationally expensive numerical models. Computational models with a high number of input parameters are fairly common in real-world applications. In such cases learning methods for building surrogate models tend to under-perform, an issue that is broadly known as the curse of dimensionality.
One way to deal with high-dimensional surrogate modelling is by first compressing the input space. Although various dimensionality reduction techniques have been suggested during the past decade, there is little work available in combining the “best of two worlds”, namely closely coupling surrogate modelling and dimensionality reduction approaches. To this end, a novel approach has been proposed that optimally combines those in a non-intrusive fashion external page[1]call_made.
The proposed approach shows superior results in terms of the surrogate model performance in the compressed space, compared to traditional methods that treat dimensionality reduction and surrogate modelling as disjoint operations.
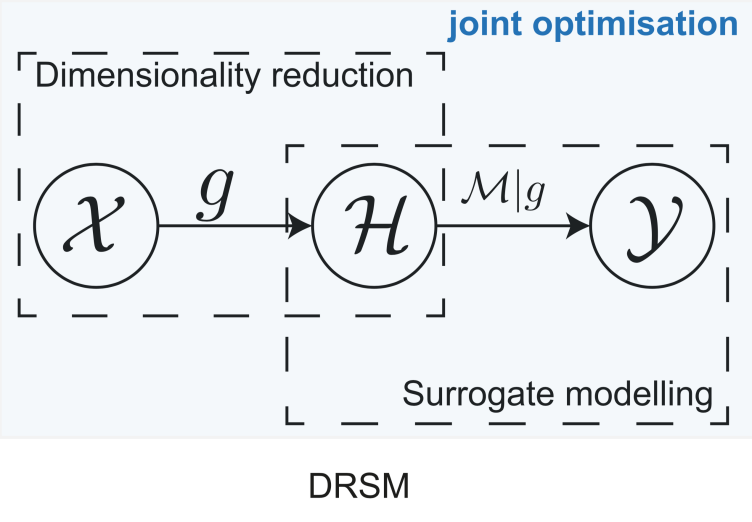
Ersatzmodelle werden häufig im Kontext der Ungewissheitsquantifizierung benutzt, um rechenintensive Computermodelle zu ersetzen. In praktischen Anwendungen haben Computermodelle häufig eine grosse Anzahl von Inputparametern. In solchen Fällen bleiben maschinelle Lernmethoden für Ersatzmodelle oft hinter den Erwartungen zurück, was auch als „Fluch der Dimensionalität“ bezeichnet wird.
Eine Möglichkeit, Ersatzmodelle für hochdimensionale Probleme zu konstruieren, ist die Kompression der Inputdaten. In den vergangenen Jahren wurden zahlreiche Dimensionsreduktions-methoden entwickelt. Allerdings gibt es nur wenige Veröffentlichungen, die „das Beste beider Welten“ – von Ersatzmodellierung und Dimensions-reduktion – kombinieren. Aus diesem Grund haben wir einen neuen Ansatz entwickelt, der diese beiden Methoden auf nicht-intrusive Weise optimal kombiniert external page[1]call_made.
Der neue Ansatz liefert bessere Ergebnisse als herkömmliche Methoden, welche Dimensions-reduktion und Ersatzmodellierung als getrennte Operationen behandeln.