Surrogate modeling and artificial intelligence in astrophysics
Principal investigators: Dr. S. Marelli, Prof. Dr. B. Sudret
Collaborators: external pageProf. Dr. R. Teyssiercall_made (Computational Astrophysics, external pageUniversity of Zürichcall_made)
Description
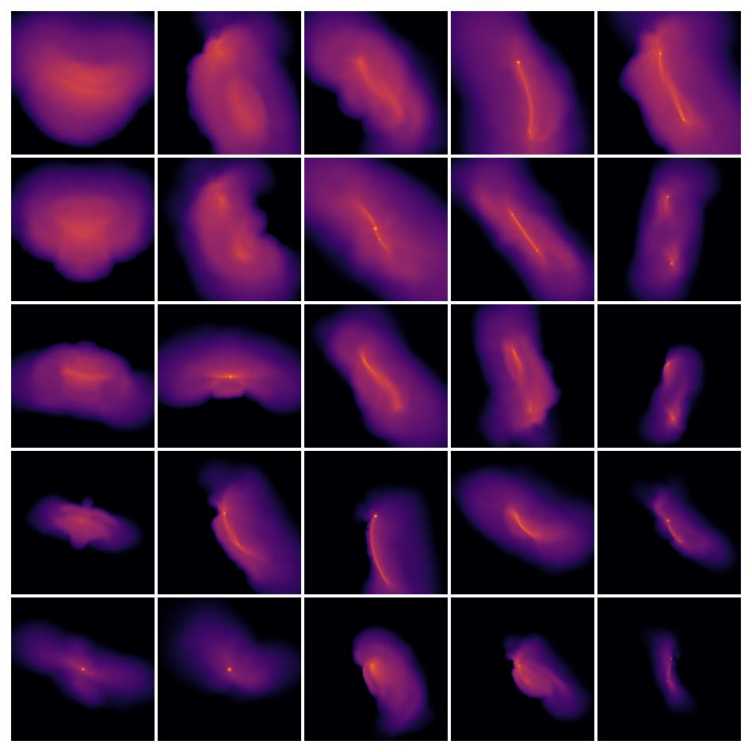
Astrophysical simulations are a powerful tool for the study of cosmological phenomena, and the origin of the universe. Due to the extreme complexity of the physics involved and the sheer size of the considered domain (i.e. the entire universe), such simulations can be remarkably expensive. It is not uncommon for a single simulation to require hundreds of thousands of node-hours to complete on state-of-the-art high-performance clusters.
In this project we demonstrate how surrogate models, a key tool in modern uncertainty quantification, can provide exceptionally accurate approximations of astrophysical simulations at the cost of relatively small set of full model runs (external pageTimpe et al., 2020call_made). The latter can then be used to assess new cosmological models at negligible costs on personal computing hardware. Moreover, we also demonstrate how they can be competitive (and in many cases more efficient than) state-of-the-art machine learning tools, such as neural networks and extreme gradient boosting trees (external pageKnabenhans et al., 2019call_made).
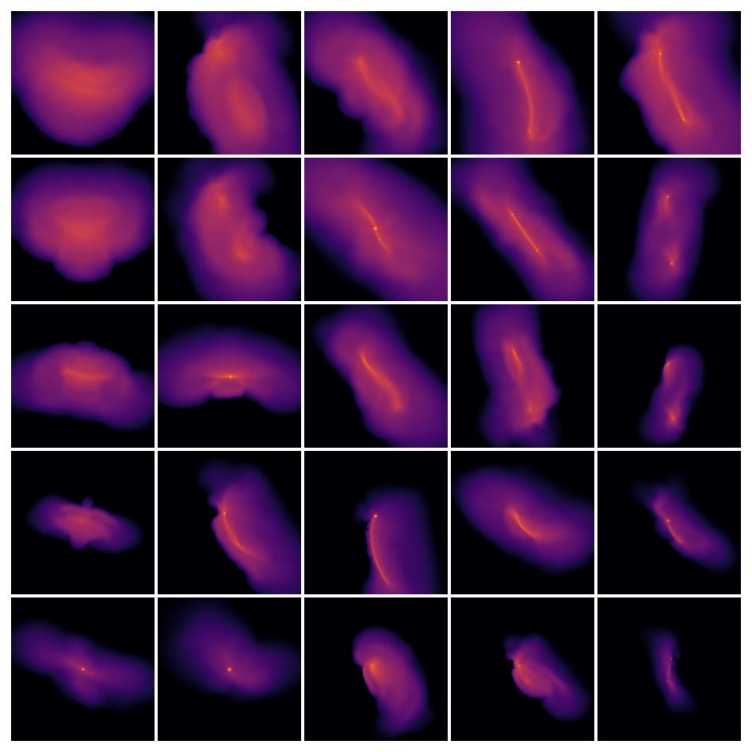
Astrophysikalische Simulationen sind ein leistungsfähiges Werkzeug für die Untersuchung kosmologischer Phänomene und des Ursprungs des Universums. Aufgrund der extremen Komplexität der untersuchten Phänomene und der schieren Größe des betrachteten Bereichs (d. h. des gesamten Universums) können solche Simulationen sehr teuer sein. Es ist nicht ungewöhnlich, dass eine einzige Simulation Hunderttausende von node-hours benötigt, um auf modernen Hochleistungsclustern berechnet zu werden.
In diesem Projekt demonstrieren wir, wie Ersatzmodelle, ein Schlüsselwerkzeug in der modernen Quantifizierung von Ungewissheiten, sehr genaue Approximationen astrophysikalischer Simulationen zu den Kosten einer relativ kleinen Menge vollständiger Modellläufe liefern können (external pageTimpe et al., 2020call_made). Letztere können dann verwendet werden, um neue kosmologische Modelle zu vernachlässigbaren Kosten auf einfacher Computerhardware zu untersuchen. Darüber hinaus demonstrieren wir, wie sie konkurrenzfähig (und in vielen Fällen effizienter als) modernste machine learning Werkzeuge, wie neuronale Netze und extreme gradient boosting trees (external pageKnabenhans et al., 2019call_made), sein können.
References