New algorithms for the robust renovation of buildings
Principal investigators: Dr. M. Moustapha, Prof. Dr. B. Sudret
Collaborators: A. Galimshina, Prof. Dr. Guillaume Habert (Chair of Sustainable Construction, ETH Zürich)
Description
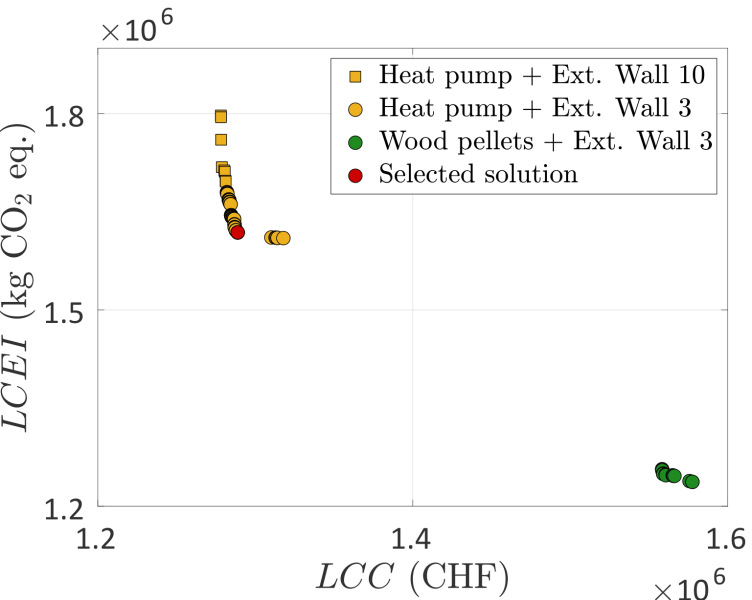
The European Union has recently pledged to renovate 35 million buildings in the next 10 years. This is motivated by the fact that buildings alone are responsible for 36% of greenhouse gas (GHG) emissions from energy in Europe. Renovating existing buildings therefore provides one of the highest potential for cost-effective GHG reduction.
Choosing an optimal renovation strategy entails repetitively carrying out life cycle analyses to estimate the life cycle cost (LCC) and environmental impact (LCEI) of a building. This task is challenging, as it requires accounting for the various sources of uncertainties affecting the building throughout its life cycle (external pageGalimshina et al., 2020call_made).
In this project, we propose to solve this problem using a multi-objective robust optimization strategy formulated by considering conservative quantiles of the two quantities of interest (LCC and LCEI). A nested two-level solution scheme combining uncertainty propagation and the non-dominated sorting genetic algorithm II (NSGA-II) (external pageDeb et al., 2002call_made), is devised. Because the associated overall computational budget would be unaffordable, an active learning strategy based on locally accurate inexpensive surrogate models, namely Kriging, is proposed. As some components of the renovation scenarios cannot be parametrized quantitatively but only qualitatively through so-called categorical variables, this methodology is adapted to account for mixed categorical-continuous variables.
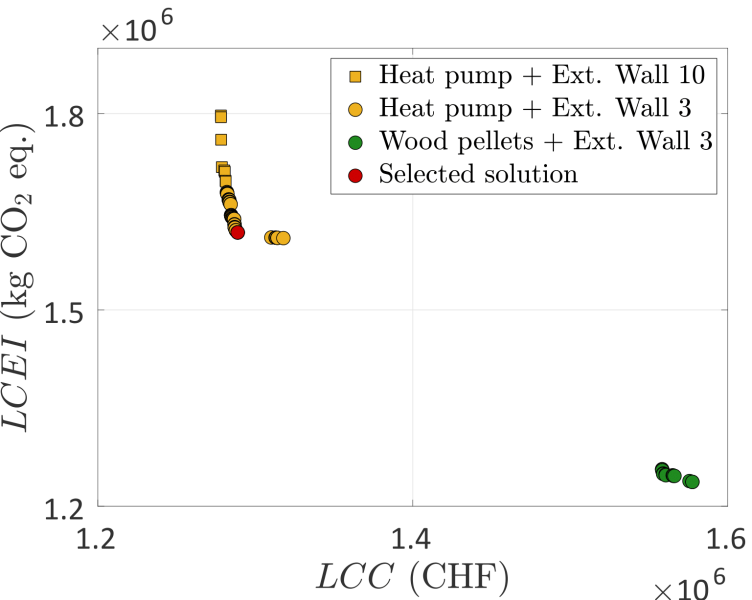
Die Europäische Union hat kürzlich zugesichert, in den nächsten 10 Jahren 35 Mio. Gebäude sanieren zu lassen. 36% der europäischen Treibhausgasemissionen (THG) im Energiesektor lassen sich auf Gebäude zurückführen, weshalb die Sanierung von bestehenden Gebäuden besonders hohes Potenzial für die kosteneffiziente Reduktion von THG bietet.
Um eine optimale Sanierungsstrategie zu entwerfen, müssen wiederholt Lebenszyklusanalysen durchgeführt werden, um die Lebenszykluskosten (LCC) und die Umweltauswirkung (LCEI) eines Gebäudes zu berechnen. Dabei müssen alle Ungewissheiten berücksichtigt werden, die das Gebäude während seines Lebenszyklus’ betreffen (external pageGalimshina et al., 2020call_made).
In diesem Projekt entwickeln wir unter Verwendung konservativer Quantile der beiden Grössen LCC und LCEI eine multi-kriterielle, robuste Optimierungsstrategie. Sie besteht aus einem geschachtelten, zweistufigen Verfahren, das Ungewissheitsfortpflanzung mit dem NSGA-II-Algorithmus (external pageDeb et al., 2002call_made) kombiniert. Weil die resultierenden Rechenkosten zu hoch wären, entwickeln wir eine aktive Lernstrategie basierend auf lokal genauen, günstigen Kriging-Ersatzmodellen. Einige Komponenten der Sanierungsszenarien können nur qualitativ, nicht quantitativ beschrieben werden (kategorische Variablen). Daher passen wir die Methodologie für den Fall gemischt kategorisch-stetiger Variablen an.
References
Deb, K., Pratap, A., Agarwal, S. and Meyarivan, T. (2002). external pageA fast and elitist multi-objective genetic algorithm: NSGA-IIcall_made, IEEE Trans. Evol. Comput., 6, 182-197.